Price floors, second price auctions and market dynamics
September 25th, 2010
One of the things that is often discussed but not often written about are the market mechanics that surround the new RTB enabled exchanges & SSPs. From a design perspective most marketplaces these days have adopted some modified form of a second-price auction. The winner of the ad impression pays the seller not his actual bid, but the second highest bid.
Second price theory works as follows: Imagine that I’m selling a Monet painting. There are people that want to buy it and each has a maximum price he’s willing to pay but of course doesn’t want to pay a penny more than he has to to get the actual painting. If I tell my buyers that they’ll only pay the second highest price then each can safely give me their maximum price because they know they’ll only pay the amount they need to to beat the next highest guy. That sounds nice right? Second price auctions maximize revenue and make everyone’s life easier and create simple and efficient markets.
The problem is, reality doesn’t seem to quite follow the theory when we look at advertising today. Take a look at the below yield curves for two publishers coming in from two different exchanges. Both of these exchanges use a second price auction model.
The way the yield curves are read is pretty simple. On the X-axis you have a CPM bid-price and on the Y-axis you have the % winrate — the probability that you will win an impression from this publisher for this ad-size if you bid this price. On the right we see a relatively logical and predictable curve — you can’t win much below $0.40, at $0.50 you win about 10% of the time and above $2.00 you will win about 70% of the time. The higher the price point, the less demand and hence the higher the winrate.
On the left you see a rather curious pattern, below $0.90 one wins nothing whereas at $1.00 you get 80-90% of all impressions. Obviously not an efficient market. In this case, the publisher has set a price floor of about $0.90 for the inventory.
This is pretty common these days in RTB — publishers are absolutely terrified about cannibalizing their rate card and are hence forcing a “premium” for RTB buyers. This is a particularly interesting case because if you look at the actual win-rates it’s pretty obvious that there is barely any demand above their actual floor… or in other words, it just ain’t worth that much. So why would a publisher set a floor and sacrifice their revenues?
What the publishers are afraid of
Fundamentally publishers are afraid that advertisers will “game” their auctions and the net result will be lower effective CPMs.
Let’s take a completely theoretical auction on Google AdExchange for a 29 year old male user seeing his third ad on a specific page about ford pickup trucks on CNN.com who has been identified as an in market cell phone shopper. Four buyers are interested in this specific impression…
- Ford buying the keywords “pickup trucks” — values the impression at a $5.00 eCPM (derived from a CPC price)
- AT&T targeting in market cell phone buyers using third party data at a $3.00 CPM
- A branded Kraft campaign that is trying to reach 29 year old males at a $2.00 CPM
In second price theory each would submit this price, Ford would win the impression with it’s $5.00 bid and pay $3.00 to match AT&T’s second price.
Here’s the problem… frequency & an abundance of supply. Users see multiple ads. Frequency is also by far the most significant variable for optimizing response to ads. Hence each buyer is only interested in hitting this user a limited number of times with their ads.
For the sake of argument, let’s assume that our 29 year old Male, Joe, is browsing a number of different articles on CNN.com and there are 10 different opportunities to deliver an ad to him. Let’s also assume that our buyers continue to bid on each and every impression. To model frequency let’s assume that after each impression delivered the advertiser will bid half as much for each subsequent impression. Under these assumptions here’s how the bids would pan out over a number of auctions:
Auction | Ford | AT&T | Kraft | Price paid |
---|---|---|---|---|
#1 | 5.00 | 3.00 | $2.00 | $3.00 |
#2 | $2.50 | $3.00 | $2.00 | $2.50 |
#3 | $2.50 | $1.25 | $2.00 | $2.00 |
#4 | $1.25 | $1.25 | $2.00 | $1.25 |
#5 | $1.25 | $1.25 | $1.00 | $1.25 |
#6 | $0.63 | $1.25 | $1.00 | $1.00 |
#7 | $0.63 | $0.63 | $1.00 | $0.63 |
#8 | $0.63 | $0.63 | $0.50 | $0.63 |
#9 | $0.31 | $0.63 | $0.50 | $0.50 |
#10 | $0.31 | $0.31 | $0.50 | $0.31 |
What we see is that for each auction the publisher’s revenue is maximized with CPMs starting at $2.50 but then very quickly dropping down to $0.63 cents.
Now here’s where theory and practice start to separate. In the above scenario, Ford pays an average of $1.72 CPM to show this user four ads. This is quite a bit higher than the average CPM and Ford decides to try a new bidding strategy to try to reduce his cost. Rather than always putting out his maximum value to the ad exchange he holds back a little bit and decides not to bid until the 6th impression.
Here’s what happens:
Auction | Ford | AT&T | Kraft | Price Paid |
---|---|---|---|---|
#1 | no bid | $3.00 | $2.00 | $2.00 |
#2 | no bid | $1.50 | $2.00 | $1.50 |
#3 | no bid | $1.25 | $1.00 | $1.00 |
#4 | no bid | $0.63 | $0.50 | $0.63 |
#5 | no bid | $0.33 | $0.50 | $0.33 |
#6 | $3.00 | $0.33 | $0.25 | $0.33 |
#7 | $1.50 | $0.33 | $0.25 | $0.33 |
#8 | $0.75 | $0.33 | $0.25 | $0.33 |
#9 | $0.38 | $0.33 | $0.25 | $0.33 |
#10 | $0.19 | $0.33 | $0.25 | $0.25 |
What you see in the above is that Ford now buys four impressions, slightly further down in the users session but for an average CPM of $0.33… 81% cheaper than were he just to submit a bid on each and every impression.
Of course this is a hypothetical situation, but it does show a point — if demand is limited then for buyers a very simple bidding strategy can have a large impact on cost and greatly increase ROI.
Let’s now imagine that the publisher realizes the advertisers are doing this and sets an artificially high floor price to try to protect his margins — $1.50. Instead of accepting a paying ad he will show a simple house ad instead.
Here’s now what the auction looks like:
Auction | Ford | AT&T | Kraft | Price Paid |
---|---|---|---|---|
#1 | no bid | $3.00 | $2.00 | $2.00 |
#2 | no bid | $1.50 | $2.00 | $1.50 |
#3 | no bid | $1.25 | $1.00 | psa |
#4 | no bid | $0.63 | $0.50 | psa |
#5 | no bid | $0.33 | $0.50 | psa |
#6 | $3.00 | $0.33 | $0.25 | $1.50 |
#7 | $1.50 | $0.33 | $0.25 | $1.50 |
#8 | $0.75 | $0.33 | $0.25 | psa |
#9 | $0.38 | $0.33 | $0.25 | psa |
#10 | $0.19 | $0.33 | $0.25 | psa |
The publisher has certainly succeeded in driving up the average Ford CPM — back up to $1.50 from the earlier $0.33. CPMs are down to $0.65 but the overall average is actually *down* from $0.71 CPM.
Here we see how the artificially high price actually ends up driving overall revenue down by limiting the number of impressions sold significantly.
Of course those of you paying attention would point out that a lower floor could potentially increase revenue over the no floor situation!
So what gives?
Direct marketers have known the above for years. This is why very few pure response driven buyers pay rate-card for the ESPN home page. What scares publishers is the idea that branded buyers could start doing the same thing. The knee-jerk reaction in this case is to set arbitrarily high floor prices on marketplace inventory to try to protect the channel conflict.
Floor prices themselves aren’t necessarily that bad. In fact, there are good reasons for setting them. First, there are brand buyers that are paying rate card for guaranteed inventory — there is no reason to expose that same inventory to those buyers on a marketplace for a much lower rate. In other cases, a publisher might just be better off displaying internal house ads rather than showing a crappy blinky offer that annoys visitors at a low CPM.
For example, imagine you’re ESPN and you have a new “Videos” section where you just started running pre-roll ads $30 CPM. If ESPN were to show the crappy blinky offer (not that they have the demand problem), they’d make $0.10 for a thousand impressions and probably risk losing a small percentage of their audience in the process. On the other hand, a benign house ad announcing the new “Videos” section of the site would both increase site-traffic and visitor loyalty, but actually generate revenue. If they get 5 clicks per thousand impressions on the house ads they’ll actually be able to net out $0.15 in advertising revenue from the 5 pre-roll impressions served on the video site (and even more if users watch more than one video).
Going back to market mechanics
Let’s go back to Market mechanics for a second. Today what we need to avoid are knee-jerk set crazy high floor prices — a floor price that is too high will simply result in lower RPMs for the publisher. Publishers must understand that there is a significant pool of demand, specifically the ROI & response driven side, that simply won’t buy the inventory for rate-card prices.
In the end it comes down to information and controls. Publishers need to understand the market mechanics and the yield that they can derive from their inventory. At the moment I’m not aware of any major marketplace providers that supply this type of information.
I have a lot of thoughts on the tools and controls a pub should have and also on how one might change market mechanics away from a true 2nd price auction to efficiently deal with this — but I think this post is getting long enough… I’ll save that for the next one (which hopefully won’t be 5 months in the making!)
Scalability Follow-Up — The challenge customers impose on innovation
April 11th, 2010
If you haven’t done so already, read this post The Challenge of Scaling an Adserver first.
I received a lot of feedback on this post, both in comments and private emails — some good, some bad. Two things were pointed out to me in relation to innovation and established players that I thought were worth a follow-up post.
When real businesses depend on you
Most startups I know release as often as they can. On the balance of testing versus finding bugs in production, most err towards production. Hell, it can take days of testing to ensure a clean release, whereas only 30 minutes in production will point out pretty much every bug in the code.
Here’s the problem — that attitude doesn’t really work once there are large public companies that depend on your platform for 100% of their entire business — often the case when you are the true adserver of record. I know, crazy idea, there are people that pay us money to run these adservers! Once a certain amount of money starts flowing through a serving system suddenly the engineering and operations teams are held to a whole new standard than before. 30 minutes of “bad data” in production can mean hundreds of thousands of dollars of lost revenue — ouch.
Generally this pressure doesn’t come until after a bad release which costs somebody a lot of money. At this point, the CEO mandates that all production releases must go through rigorous testing to ensure that such a catastrophic event will absolutely never happen again. Immediately job postings will go out for more QA engineers and very quickly every release has to go through several steps of vetting and testing before it is deemed ok to release. Very quickly, cultures change to focus less on features and more on stability. As we all know… stability is the evil enemy of change. Change, sadly, is a requirement for innovation.
Invisible Features
The second thing I forgot to mention in my last post is the concept of “invisible features”. Running a simple adserver with little volume there are a very large number of “off the shelf” components that you can use that simply work at low scale.
Take for example server-side cookies. Imagine I’m the small startup discussed in our last post. I’m seeing about 100k unique users a day with my adserver, for a total of about 1M/month. I store about 1kb worth of data per user which means I have about a gigabyte of total data to store. I’m only doing about 3000 ads per second, so I buy myself a nice new shiny Dell server, install MySQL and use that as my server-side data store. Every few hours or so I use mysqldump to make a full copy of my 1GB of data and copy that to a central storage server. I’ve got gigabit ethernet so it only takes about 12.5 seconds to make a full backup of my data.
Compare that to an enterprise adserver… that’s got a few billion ads a day with 150M unique users/month, with 3kb of data each. Not only that, but enterprise active customers require redundant datacenters to meet SLAs — this means all data has to be available on both the east and west coasts — at the same time. This means the enterprise adserver has to store 450GB of data in two datacenters, and be able to read/write to this data 100s of thousands of times per second. Guess what MySQL will do in this situation?
KABLOOOEY!
I won’t dive into all the details, but suffice to say that just backing up 450GB of data will take over an hour and a half on a GigE connection — compare that to the 12.5 seconds it takes at small scale and you probably get an idea of how hard of a problem it is to keep 450GB of data in sync across the country. There is no off the shelf solution that addresses this problem. What that means is that instead the engineering teams now need to focus significant energy and resources into building a mass distributed primary-key value store.
Functionally there is little to no difference to the end-user of the adserver of the two different systems. Both store a certain amount of data on a user… the difference is one system scales and requires a significant amount of effort while the other doesn’t. This is what I would call an “invisible feature”. It’s one of the things that one has to do to be a viable scalable adserving company but one that goes utterly unnoticed by customers (assuming everything works).
Server side cookies aren’t the only one of these systems that can be found “off the shelf” at low volumes but break when we start talking billions. Data pipelines, data warehouses, ETL systems, operational systems that can manage 100s of servers at a time — and this isn’t even to mention all the supporting hardware an infrastructure — core switches, load-balancers, CDNs and ISPs — each items that start to fail at billions and require upgrades and custom integrations to function properly at scale.
How do you know?
One of the things that’s so challenging in this market is that few of the new technology companies has had to deal much with the scale problem. So as a buyer of an adserving system, how do you know that it’ll work? If both company A and company B have server-side cookies, how do you pick the one that’ll work at scale? That’s the problem… if neither is doing serious volume, who knows! More on that in another blog post.
PS: There’s a great paper on Amazon’s Dynamo Store — a similar technical problem to the one most adservers face on building server-side cookie stores. It’s a great read if you’re curious as to the problems one needs to solve at scale.
The Challenge of Scaling an Adserver
April 4th, 2010
So much of our time these days is spent talking about all the new features & capabilities that people are building into their adserving platforms. One component often neglected in these conversations is scalability.
A hypothetical ad startup
Here’s a pretty typical story. A smart group of folks come up with a good idea for an advertising company. Company incorporates, raises some money, hires some engineers to build an adserver. Given that there are only so many people in the world who have built scalable serving systems the engineering team building said adserver is generally doing this for the first time.
Engineering team starts building the adserver and is truly baffled as to why the major guys like DoubleClick and Atlas haven’t built features like dynamic string matching in URLs or boolean segment targeting (eg, (A+B)OR(C+D)). Man, these features are only a dozen lines of code or so, let’s throw them in! This adserver is going to be pimp!
It’s not just the adserver that is going to be awesome. Why should it ever take anyone four hours to generate a report, that’s so old school. Let’s just do instant loads & 5-minute up to date reporting! No longer will people have to wait hours to see how their changes impacted performance and click-through rates.
The CEO isn’t stupid of course, and asks
“Is this system going to scale guys?”
“Of course”
Responds the engineering manager. We’re using this new thing called “cloud computing” and we can spool up equipment near instantly whenever we need it, don’t worry about it!
And so said startup launches with their new product. Campaign updates are near instant. Reporting is massively detailed and almost always up to date. Ads are matched dynamically according to 12 parameters. The first clients sign up and everything is humming along nicely at a few million impressions a day. Business is sweet.
Then the CEO signs a new big deal… a top 50 publisher wants to adopt the platform and is going to go live next week! No problem, let’s turn on a few more adservers on our computing cloud! Everything should be great.. and then…
KABLOOOOOOOOEY
New publisher launches and everything grinds to a halt. First, adserving latency sky-rockets. Turns out all those fancy features work great when running 10 ads/second but at 1000/s — not so much. Emergency patches are pushed out that rip out half the functionality just so that things keep running. Yet, there’s still weird unexplainable spikes in latency that can’t be explained.
Next all the databases start to crash with the new load of added adservers and increased volume. Front-end boxes no longer receive campaign updates anymore because the database is down and all of a sudden nothing seems to work anymore in production. Reports are now massively behind… and nobody can tell the CEO how much money was spent/lost in over 24 hours!
Oh crap… what to tell clients…
Yikes — Why?
I would guess that 99% of the engineers who have worked at an ad technology company can commiserate with some or all of the above. The thing is, writing software that does something once is easy. Writing software that does the same thing a trillion times a day not quite so much. Trillions you ask… we don’t serve trillions of ads! Sure, but don’t forget for any given adserver you will soon be evaluating *thousands* of campaigns. This means for a billion impressions you are actually running through the same dozen lines of code trillions of times.
Take for example boolean segment targeting — the idea of having complex targeting logic. Eg, “this user is in both segments A and B OR this user is in segments C and D”. From a computing perspective this is quite a bit more complicated than just a simple “Is this user in segment A”? I don’t have exact numbers on me, but imagine that the boolean codetakes about .02ms longer to compute on a single ad impression when written by your average engineer. So what you say, .02ms is nothing!
In fact, most engineers wouldn’t even notice the impact. WIth only 50 campaigns the total impact of the change is a 1ms increase in processing time — not noticeable. But what happens when you go from 50 campaigns to 5000? We now spend 100ms per ad-call evaluating segment targeting — enought to start getting complaints from clients about slow adserving. Not to mention the fact that each CPU core can now only process 10 ads/second versus the 1000/s it used to be able to do. This means to serve 1-billion ads in a day I now need 3,000 CPU cores at peak time –> or about 750 servers. Even at cheap Amazon AWS prices that’s still about $7k in hosting costs per day.
Optimizing individual lines of code isn’t the only thing that matters though. How systems interact, how log data is shipped back and forth and aggregated, how updates are pushed to front-end servers, how systems communicate, how systems are monitored … every mundane detail of ad-serving architecture gets strained at internet scale.
Separating the men from the boys…
What’s interesting about today’s market is that very few of the new ad technologies that are entering the market have truly been tested at scale. If RTB volumes grow as I expect they will throughout this year we’ll see a lot of companies struggling to keep up by Q4. Some will outright fail. Some will simply stop to innovate — only a few will manage to continue to both scale and innovate at the same time.
Don’t believe me? Look at every incumbent adserving technology. DoubleClick, Atlas, Right Media, MediaPlex, OAS [etc.] — of all of the above, only Google has managed to release a significant improvement with the updated release of DFP. Each of these systems is stuck in architecture hell — the original designs have been patched and modified so many times over that it’s practically impossible to add significant new functionality. In fact, the only way Google managed to release an updated DFP in the first place was by completely rebuilting the entire code base from scratch into the Google frameworks — and that took over two years of development.
I’ll write a bit more on scalability techniques in a future post!
New York Times article on RTB
March 11th, 2010
Sorry for a bit of self promotion, but, Stephanie Clifford from the New York Times just published a great story on real-time bidding and how AppNexus & eBay have worked together over the past year to dramatically increase ROI with Real-Time buying from companies like Google:
Instant Ads Set the Pace on the Web.
Scary, I’ve been trying to explain to my mother what I do for years, but I think she’ll finally get it after reading this.
Ugh, commenting has been broken
March 4th, 2010
Apologies to all that posted comments on the blog the past 2 weeks, appears that my comment spam filter has marked every comment submitted as spam! I went through a good chunk just now but have about 1500 spam comments to weed through so there are probably a few I’ve missed.
I still have to figure out why the spam filter is broken, but please be aware that comments might take a few hours to show up but aren’t going into a black hole!
-Mike
PS: Normally comments are auto-approved unless they contain too many links …
Everybody’s a DSP, wait, what’s a DSP?
February 26th, 2010
Unless you’ve been living under a rock you’ve heard the term ‘DSP’ — “Demand Side Platform” thrown around like no other. The term has hit such a buzz that there is almost no meeting that doesn’t start with — “Wait, are you a DSP? What is a DSP? Is ____ a DSP? Are you working with a DSP? Which DSP should I work with?”.
Let’s start with some history. I’m not quite sure who coined the term originally but it was primarily used to describe cross exchange buying desks & bid management solutions like Invite, Turn & DataXu. We at AppNexus even briefly described ourselves as a DSP — that was of course when the ‘Platform’ was still a part of DSP — more on that in a bit.
Since then, agencies started allocating budgets away from “networks” and towards “DSPs”…
Wait… what?
You got it –agencies, eager to cut into the hefty margin networks take, started to allocate budgets towards DSPs for exchange buying. What’s of course ironic, is that the typical relationship between an agency and a DSP is certainly not a “platform” relationship but a simple media IO. Early versions of the platforms weren’t (and many still aren’t) mature and the only way they could make them work is by having people manually traffic and optimize campaigns on ad exchanges. Although the spirit of the relationship was one of a platform optimizing media across exchanges the reality is that it is primarily a service driven offering… something which practically looks very similar to a network with a slightly more defined box of inventory and in some cases clearer rules & margins.
Networks of course become massively threatened when their IO budgets got cut in favor of DSPs & Exchange Buying. Now if you’re a network, what do you do? You just rebrand yourself as a DSP or launch a new DSP arm of the business! Voila… now you can go right back to the exchange and say — “Oh, we do that too!”. Now every ad-network is suddenly a DSP…. Of course the response from the first set of DSPs has been to quickly try to define DSPs as “not a network”. You can read two examples on AdExchanger here: Zach Coelius @ Triggit, Nat Turner @ Invite.
Now the majors (Google/Yahoo/MSFT) of course see their direct relationships with the agencies being marginalized by this new “DSP” concept. So what do you do… that’s right, you become a DSP!! Yahoo has already announced this strategy and Google has been rumored to buy a DSP — Microsoft can’t be far behind.
There you have it… who is a DSP? Everybody. The problem is that in this whole process the ‘P’ of ‘DSP’ has disappeared — where is the platform– it just seems to be anybody who plays on the Demand Side — technology vendors, ad-networks & brokers, portals and a few hybrid tech/network companies. Platforms draw valuations, therefore everybody is a platform. The thing is that platform implies that people can build technology on top of your technology. Of the umpteen companies calling themselves a ‘DSP’, how many can say that there is technology being built on top of them? How many have open APIs that you can integrate with?
Here is my proposal… let’s retire the term DSP. It’s loaded, and effectively doesn’t mean anything anymore. Instead, let’s talk about Display Engine Marketing (DEM) and ad technology vendors. DEM companies are ones that will take your media $ and optimize it for you across aggregators of Display. This is the media relationship. Adserving & RTB technology vendors are ones that will license a technology — which may or may not be a platform on it’s own — to integrate with supply aggregators and help run a DEM business. Of course going to be DEM companies that build their own and some that license others, that’s expected in this world. Similarly there are going to be technology vendors that have their own in house DEM teams (*cough* dem==ad network *cough*) that will take an IO and run the media on your behalf.
In the end I want to point you back to an old post I wrote: I don’t care who you say you are, what do you DO?. The next time someone says they are a DSP — respond with — “That’s great, but what do you actually do?”
RTB Part III — Cookies & User Data
February 22nd, 2010
Cookie Matching.
A number of people have asked about how cookie matching works in the RTB world. Everybody today relies on cookies to store information about a user — which ads he’s seen, what sites he’s been to, which behavioral segment he’s in — all the good stuff. Without cookies, we can’t frequency cap, we can’t remarket, we can’t track, basically, advertising becomes pretty useless!
Now the way browsers work, cookies are tied to a domain. Say an ad-exchange has ad tags on a page under ‘ad.exchange.com’. When a browser requests an ad from the exchange, it only passes along the cookie data that’s stored inside that domain name. This means that the exchange has zero insight into whatever data the bidder might have collected under ad.bidder.com.
So why is this a problem? Well, if the exchange is going to do a server-side bid request to the bidder, it’s pretty hard for the bidder to make a decision about what ad to serve if it has no access to either frequency or behavioral data!
An Example. Bidder is running a behavioral campaign for marketer “marketer.com”. Bidder has a pixel on the marketer’s site, it’s internal ID for the pixel is 27. Each time this pixel fires the bidder ads a cookie to it’s domain ‘ad.bidder.com’, ‘segment=27′. The bidder now wants to buy as much inventory as possible for this remarketing segment across exchanges. Bidder has a user-id, ‘ABC’ for this cookie.
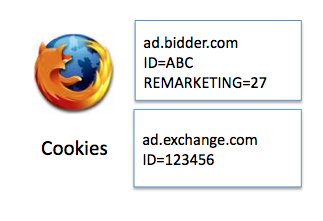
Exchange, ‘exchange.com’ wants the bidder to be able to buy as much inventory as possible. Hence the exchange wants the bidder to be able to use all remarketing segments to buy. Problem is (as mentioned above), they’re using separate cookie domains. So what needs to happen is that the exchange and the bidder need to synchronize their domains so that when the bidder receives a real time bid request he can look-up the information associated with that user.
Fundamentally the logic for synchronizing IDs is pretty simple. The exchange drops a pixel on a page somewhere which points to a bidder’s domain and a simple usersync call. When the call hits the bidder, it reads in it’s cookie ID, redirects off to the exchange passing it’s ID in the querystring so that the exchange can read and store it into it’s cookie space.
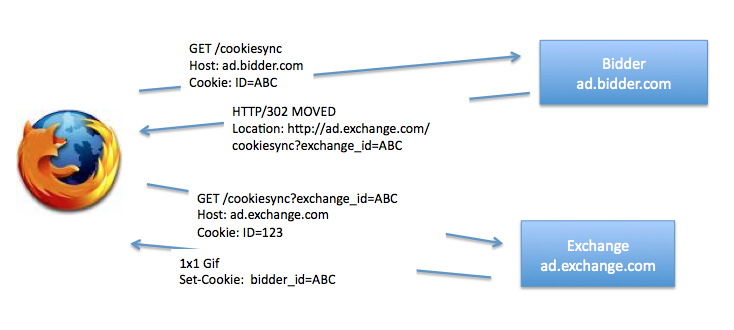
The above diagram shows this process. First a pixel is dropped that hits the bidder domain. The bidder receives this call and reads in it’s cookie ID of ‘ABC’. Bidder then redirects the user to get a request from the exchange under ad.exchange.com, passing in the id ‘ABC’ in the querystring. The exchange now receives a call, reads in from the cookie that his ID is 123, and can now map that his user 123 maps to bidder’s user ABC.

Now user IDs are synchronized, the exchange and the bidder can talk about a single user in the same manner. Note that in the above example I noted that the exchange is storing the ID for the bidder. Admeld, PubMatic & the like all work like this. Google does it slightly differently and instead requires the bidder to store the mapping on his end — effectively just the reverse of the above process.
RTB Serving Speed
October 18th, 2009
One of my readers posted the following comment on my first post on RTB:
In your second diagram you show the interaction between the publisher adserver and multiple networks. Does this potentially multiple source back and forth not slow down the adserving in the same way a series of dumb redirects would? Especially when you consider that presumably if Network 1 came back with the best price out of 3 or four networks, once the publisher ad server knew that it would need to go back to it and request the actual ad again. It would be interesting to see some realistic HTTP traces for this stuff.
This is indeed a great question. Technically it looks like there are the same # of requests going back and forth in RTB versus a traditional ad-call. Although this is the case, RTB is going to be significantly faster… and here’s why.
Technically a browser downloading content from an adserver is a five step process:
* DNS lookup of the adserver domain name
* Establishment of a TCP connection
* Requesting content
* Acknowledge of request & sending back content
* Terminating the TCP connection
Assume for this case that a DNS lookup takes about 100ms. Each of these steps requires a number of packets to go from the local computer up to the adserver and a series of response packets. Here’s the # required for each step:
* TCP Connection: Two packets up, and one packet down (SYN, SYN-ACK, AKC)
* Requesting content: One packet up (minimum)
* Request acknowledgement and content: One packet down (minimum) & one packet up
* Terminating the connection: One packet
So the minimum number of packets sent back and forth is 7. If the latency from an end-user is 50ms to the adserver, this means it will take *at least* 450ms (100ms DNS + 350ms ad-request) to request the ad.
Now you’d think this would be the same for real-time, but it’s not! There are three reasons a request between two serving systems is much faster:
* Better connectivity — Adservers are hosted in datacenter that generally have much better internet connectivity than the average end-user. This means lower latency between the two adserving systems.
* No DNS lookup — The RTB system can cache DNS lookups for all RTB partners, effectively removing this 100ms.
* Persistent TCP connections — Any intelligent RTB integration would use persistent TCP sessions between the sell and buy side systems. This means a connection is established once and reused thousands of times after that.
With the above three, here’s how requesting a “bid” looks from sell to buy side:
* Requesting content: One packet
* Acknowledge of request & sending back content: One packet
So assume 25ms latency between systems (rather than 50) and the minimum time for an RTB request between systems is only 50ms compared to the 450ms it would take for an actual end-user or 9 times faster. The slower the end users connection and the faster RTB will be.
Conclusion — yes, adserving individual requests becomes a little bit slower but the removal of redirects makes the overall process signficantly faster.
For those technically curious, here’s are tcpdumps that prove this.
Browser to adserver:
15:45:04.380042 IP 10.0.1.31.59541 > 8.12.226.77.http: Flags [S], seq 50484529, win 65535, [...] 15:45:04.397395 IP 8.12.226.77.http > 10.0.1.31.59541: Flags [S.], seq 661028066, ack 50484530 [...] 15:45:04.397529 IP 10.0.1.31.59541 > 8.12.226.77.http: Flags [.], ack 1, win 65535, length 0 15:45:04.397831 IP 10.0.1.31.59541 > 8.12.226.77.http: Flags [P.], seq 1:1288, ack 1, win 65535, length 1287 15:45:04.424466 IP 8.12.226.77.http > 10.0.1.31.59541: Flags [.], seq 1:1461, ack 1288, win 62780, length 1460 15:45:04.424472 IP 8.12.226.77.http > 10.0.1.31.59541: Flags [P.], seq 1461:1543, ack 1288, win 62780, length 82 15:45:04.424546 IP 10.0.1.31.59541 > 8.12.226.77.http: Flags [.], ack 1543, win 65535, length 0
Adserver to adserver with persistent connections:
20:00:10.709152 IP 64.208.137.8.41096 > 8.12.226.77.80: . ack 1023 win 7154 20:00:10.754844 IP 8.12.226.77.80 > 64.208.137.8.41096: P 1023:2045(1022) ack 501 win 62780
Microsoft sues the bad guys
September 19th, 2009
Check out the post by Tim Cranton on theMicrosoft Blog about five suits he just filed against a variety of malware purveyors.
Kudos to Microsoft for taking action! The wild-wild west of display advertising is slowly growing up.
RTB Part II: Supply supply supply!
September 19th, 2009
Please check out my last post on RTB first. Since this last post, a pretty big announcements has hit the wire. Namely, Google has announced Ad Exchange 2.0. Most significantly:
One of the platform’s key features is the ability for ad networks and agency buyers to bid on inventory in real-time, letting them zero in on impression attributes such as geographic location or the presence of advertiser cookies before placing a bid. Yahoo’s Right Media ad exchange does not currently offer bidding in real-time, though it is available through some smaller ad marketplaces.
That’s right folks — Google’s real time exchange is coming. In this post we’ll talk about who is jumping on the RTB band-wagon on the supply side, and some implications this is going to have on the industry.
Ok Who’s In
Ok, so Google is doing it? Who else? Over the past few months pretty much any aggregator of supply has launched, announced or started work on some sort of RTB capability. All major exchanges — Yahoo’s Right Media, Microsoft’s AdECN and Google’s AdEx have RTB integrations in the works. Of the pub aggregators, AdMeld & PubMatic are live and Rubicon is actively working on a solution. As mentioned, FAN has been live with Myspace inventory for a while and there are a number of other parties, such as ContextWeb, AdBrite and OpenX, entering the space. The short summary is, over the next 12 months we can expect billions of daily impressions hitting hundreds of millions of unique users to become available RTB.
Death of the Traditional Ad Network
This is going to have huge implications for the display advertising space — primarily, the traditional ad-network model is on it’s last legs. Most traditional ad-networks today thrive because they have large business development teams that have developed deep relationships with supply. Ad.com, Specific Media & ValueClick all have large publisher bases that they rep to agencies. This posed a large difficult hurdle for new networks to overcome and effectively created a catch-22 for any new network entering the space. To get media dollars a network needs reach, but to get publisher deals a network needs media dollars.
This all changes when there are billions of biddable impressions out there. In this new world, any new network has instant access to the reach that historically would take years to build up. Now anybody can walk into an agency and claim to have a reach of over 100 million unique users.
Now of course this isn’t totally new. Right Media opening up Yahoo inventory to the world back in 2007 started this process and a number of companies have managed to start very succesful network (or “exchange desk”) businesses on this platform. AdECN, AdEx, Admeld, PubMatic and Rubicon take this to a new level as this opens up MSN, the DFP publisher base and the majority of the Comscore 1000 list!
Can Technology Finally Win?
I’ve written in the past about the plight of the ad-technology startup. The short summary is this — technology is great, but the lions share of revenue today comes from media not tech.
As access to inventory becomes commodity marketers and brands will inevitably start focusing on results over the ability to simply spend the budget. With everyone on an even playing field it’ll be easy for a marketer to compare the results from one buying network to the next — which means technology will finally matter.
There are already a number of successful technology focused startups who focus on exchange buying — and a couple that are simply building cross-exchange buying tools. Expect this to become the next hot space for startups in the advertising world.
Behavior behavior behavior!
The traditional problem with behavioral buying, whether remarketing or using third party data provided by companies like IXI, Exelate or Blue Kai, has always been reach. We all know that remarketing works wonders and has amazing ROI, but unless you can actually find your users on the web it’s hard to spend a significant amount of money this way.
With billions of impressions that of course changes — the probability of finding a user across the many RTB platforms becomes easier and easier and hence the actual required reach of any given behavioral segment becomes smaller and smaller. This in itself is going to make data-focused businesses more feasible and also open up a world of possibilities of highly targeted and focused media-campaigns and very granular behaviors.
Next Up
Ok, enough RTB for the day. Next up –> demand demand demand! Who are the new players that are taking advantage of this new RTB revolution and innovating both from a business model & technology perspective.